Unit Testing Spark Jobs
I went down the rabbit hole of Spark unit testing. As it’s uncharted territory, you find a lot of conflicting opinions. I decided to hack my way through it. This post is a summary of my thoughts and findings. I try to go into the process I went through to resolve the problems encountered.
To escape the theory, I will use concrete examples throughout the article. The data represent the number of flights count
, from origin
countries to destination
countries. It has the following structure:
| Destination | Origin | Count | |-------------|---------|-------| | Morocco | Spain | 3 | | Morocco | Egypt | 5 | | France | Germany | 10 | | ... | ... | ... |
The structure is mindlessly inspired by the Spark: The Definitive Guide’s Code Repository. You find the code here.
Before going into the testing section, the tools to use, the project’s structure, and the reasoning behind a testable Spark job. First things first, the structure of the project:
+-- project | +-- build.properties | +-- plugins.sbt +-- src | +-- main | +-- test +-- .gitignore +-- .scalafix.conf +-- .scalafmt.conf +-- LICENSE +-- build.sbt +-- README.md
Dependencies
Starting with Spark dependencies:
// build.sbt
libraryDependencies ++= Seq(
"org.apache.spark" %% "spark-core" % sparkVersion,
"org.apache.spark" %% "spark-sql" % sparkVersion,
"org.apache.spark" %% "spark-hive" % sparkVersion % provided
)
Loading configuration
For configuration loading, you can use PureConfig.
Add the following to your build.sbt
:
// build.sbt
// https://github.com/pureconfig/pureconfig
libraryDependencies += "com.github.pureconfig" %% "pureconfig" % "0.14.0"
Then create a /src/main/resources/reference.conf
file:
# reference.conf
settings {
database = "dev"
}
To be able to load the configuration, you need to create a Settings
case class:
// src/main/scala/me/ayoublabiad/common/Settings.scala
package me.ayoublabiad.common
case class Settings(
database: String
)
Then you can load the configuration wherever you need it:
import me.ayoublabiad.common.Settings
import pureconfig.ConfigSource
val settings: Settings = ConfigSource.default.loadOrThrow[Settings]
Then you can pass the settings
object to your Spark job.
FlightsJob.process(spark, settings)
Testing tools
For testing, you will be using:
// build.sbt
// https://mvnrepository.com/artifact/com.fasterxml.jackson.module/jackson-module-scala
libraryDependencies += "com.fasterxml.jackson.module" %% "jackson-module-scala" % "2.13.4"
// https://mvnrepository.com/artifact/org.scalatest/scalatest-flatspec
libraryDependencies += "org.scalatest" %% "scalatest-flatspec" % "3.2.14" % "test"
// https://github.com/MrPowers/spark-fast-tests
libraryDependencies += "com.github.mrpowers" %% "spark-fast-tests" % "0.23.0" % "test
Scalatest
for creating unit tests, Spark-fast-tests
for comparing DataFrames, and jackson
for parsing JSON.
Starting with Scalatest
. It’s a testing framework for Scala. It’s a good fit for Spark as it’s a Scala library.
Plugins
To make your life easier, you can use the following plugins:
// project/plugins.sbt
addSbtPlugin("ch.epfl.scala" % "sbt-scalafix" % "0.9.24")
addSbtPlugin("org.scalameta" % "sbt-scalafmt" % "2.4.0")
Scalafmt
Scalafmt for code formatting. You can enable it in your IntelliJ IDEA by going to: CTRL + ALT + S
> Editor
> Code Style
> Scala
> Formatter
Change it from IntelliJ
to Scalafmt
. Then add the following configuration to the root directory of your project:
# .scalafmt.conf
version = "2.7.5"
# Check https://github.com/monix/monix/blob/series/3.x/.scalafmt.conf for full configuration
The shortcut to format your code in IntelliJ IDEA is CTRL + ALT + L
.
Scalafix
Scalafix is a linting and refactoring tool. Add the following configuration to the root directory of your project:
# .scalafix.conf
# https://spotify.github.io/scio/dev/Style-Guide.html
rules = [
RemoveUnused,
LeakingImplicitClassVal
]
Add the following to your build.sbt
:
// built.sbt
// https://scalacenter.github.io/scalafix/docs/users/installation.html
inThisBuild(
List(
scalaVersion := "2.11.12",
semanticdbEnabled := true, // enable SemanticDB
semanticdbVersion := scalafixSemanticdb.revision // use Scalafix compatible version
)
)
scalacOptions ++= List(
"-Ywarn-unused"
)
To run the rules, execute this command:
sbt scalafixAll
Project structure
This might be the point of divergence. Each team has its own structure adapted to its specific needs. Here are a couple of things to consider:
scala +-- me.ayoublabiad | +-- common | | +-- SparkSessionWrapper.scala | +-- io | | +-- Read.scala | | +-- Write.scala | +-- job | | +-- flights | | | +-- FlightsTransformation.scala | | | +-- FlightsJob.scala | | +-- Job.scala | +-- Main.scala
common: Package for shared classes between jobs. A
Utils
class containing helper functions can be added here.io: Package for reading and writing data. A
Read
andWrite
object can be added here.job: Package for jobs. Each job should be in its own package. A
Job
trait can be added here.- The transfrom functions could have one of the following signatures:
def function1(dataFrame: DataFrame): DataFrame // or def function2(column: Column): Column // or even def function3(columnName: String): Column
This way, you can compose the transformations in a more functional way:
val result = dataFrame .transform(function1) .withColumn("newColumnName", function2(col("oldColumnName"))) .withColumn("newColumnName", function3("oldColumnName"))
You can also test the transformations in isolation.
- The job package where you group the three phases: reading, transforming, and writing. In its simplest form, it should look something like this:
import com.typesafe.config.Config import me.ayoublabiad.io.Read.readTableFromHive import me.ayoublabiad.io.Write import me.ayoublabiad.job.Job import org.apache.spark.sql.functions.{col, lit} import org.apache.spark.sql.{DataFrame, SparkSession} object FlightsJob extends Job { override def process(spark: SparkSession, config: Config): Unit = { val db: String = config.getString("settings.database") val flights: DataFrame = readTableFromHive(db, "flights") val destinationsWithTotalCount = FlightsTransformation.getDestinationsWithTotalCount(flights) Write.writeTohive(spark, filteredDestinations, db, "flights_total_count") } }
Main: The entry point of your job. It’s responsible for loading the configuration and running the jobs.
We will spend the rest of the article on the FlightsJob
and FlightsTransformation
classes.
A case for testing Spark jobs
Spark job is composed of three phases:
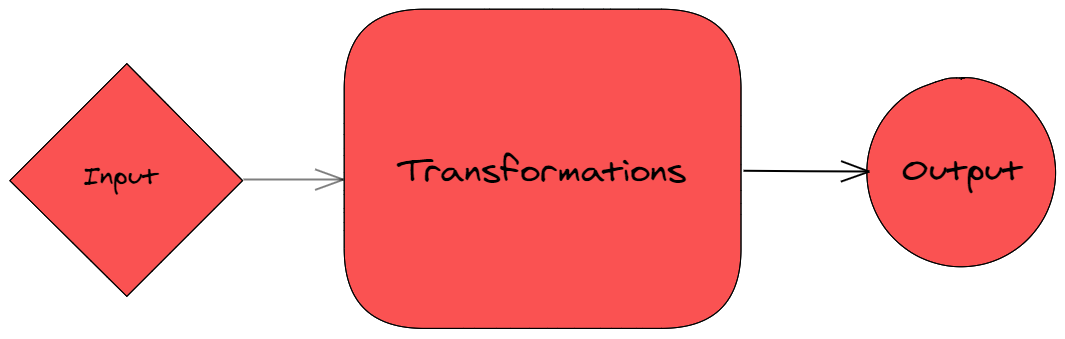
You read your data, transform it, and write it. While you aren’t trying to test Spark itself, you can test the transformations. You are trying to answer the question: “Does my transformation work as expected?”
Let’s concretize those ideas with an example. Based on the flight table described before, you want to get the total number of flights per destination. You can write the following transformation:
import org.apache.spark.sql.functions.sum
def getDestinationsWithTotalCount(flights: DataFrame): DataFrame =
flights
.groupBy("destination")
.agg(sum("count").alias("total_count"))
Assuming that you need the origin
country column to appear in the result, you can write the following transformation using Window
:
import org.apache.spark.sql.expressions.Window
import org.apache.spark.sql.functions.count
def addTotalCountColumn(flights: DataFrame): DataFrame = {
val winExpDestination = Window.partitionBy("destination")
flights
.withColumn("total_count", count("count").over(winExpDestination))
}
Is this function worthy of testing? Probably not if:
- You remember that the boundaries of
Window
by default areUnboundedPreceding
andUnboundedFollowing
; - If you noticed that, the function
count
is used instead ofsum
.
While this is a simple example, you can imagine that the transformations can be more complex. You can also imagine that the transformations can be reused in other jobs. In this case, you want to make sure that the transformations work as expected. I think that Raymond Hettinger talk about The Mental Game of Python is relevant in this context. We can only keep a limited amount of information in our minds. We need to be able to trust our code.
Although the earlier implementation is correct, it’s not the most efficient. You can rewrite it using groupBy
and sum
:
def getDestinationsWithTotalCount(flights: DataFrame): DataFrame =
flights
.groupBy("destination")
.agg(
collect_list("origin").alias("origins"),
sum("count").alias("total_count"))
Or is it? But you get the idea.
Another example to take a look at is getting a value from a column. The tests for this function would be:
"getParamFromTable" should "return the param if it exists" in {
val input: DataFrame = createDataFrame(
obj(
"minFlightsThreshold" -> 4
)
)
val threshold: Long = getValueFromDataFrame(spark, input, "minFlightsThreshold")
assert(threshold == 4)
}
"getParamFromTable" should "throw an Exception if the threshold doesn't exist" in {
val input: DataFrame = Seq.empty[Long].toDF("minFlightsThreshold")
val caught =
intercept[Exception] {
getValueFromDataFrame(spark, input, "minFlightsThreshold")
}
assert(caught.getMessage == "Threshold not found.")
}
The implementation looks something like this:
def getValueFromDataFrame(dataFrame: DataFrame, columnName: String): Long =
Try(dataFrame.select(columnName).first().getLong(0)).getOrElse(throw new Exception("Threshold not found."))
This function can go wrong in different ways. You can forget to check if the column exists. You can also forget to check if the column is empty. You can also forget to check if the column is of type Long
.
If we agreed that we should test Spark jobs, How to go about it?
How to test Spark jobs?
Let’s create Spark Session first:
// https://github.com/MrPowers/spark-fast-tests/blob/master/src/test/scala/com/github/mrpowers/spark/fast/tests/SparkSessionTestWrapper.scala
trait SparkSessionTestWrapper {
lazy val spark: SparkSession =
SparkSession
.builder()
.master("local[1]") // One partition One core
.appName("SparkTestingSession")
.config("spark.sql.shuffle.partitions", "1")
.getOrCreate()
}
To create a Spark DataFrame, you use one of the following methods:
Using .toDF
After importing spark.implicits._
you do the following manipulation:
val input: DataFrame = Seq(
("morocco", "spain", 3),
("morocco", "egypt", 5),
("france", "germany", 10)
).toDF("destination", "origin", "count")
This method is convenient and fast. But it has some limitations. You can’t create a DataFrame with a null
value. You can’t create a DataFrame with a StructType
column. You can’t create a DataFrame with an ArrayType
column. And it lacks readability as soon as you have more than 3 columns.
We need a more flexible and readable way to create DataFrames. What’s the most intuitive way of representing a key-value pair? A Map
! Let’s create a Map
and convert it to a DataFrame:
From JSON to DataFrame
The idea is to represent each row as a Map
. It might be verbose but it’s readable. You can use the following function to convert a Map
to a DataFrame
:
import org.apache.spark.sql.DataFrame
import spark.implicits._
import org.json4s.DefaultFormats
import org.json4s.jackson.Json
def createDataFrame(data: Map[String, Any]*): DataFrame =
spark.read.json(Seq(Json(DefaultFormats).write(data)).toDS)
Then to create a DataFrame:
val input: DataFrame = createDataFrame(
Map(
"destination" -> "morocco",
"origin" -> "spain",
"count" -> 3
),
Map(
"destination" -> "morocco",
"origin" -> "egypt",
"count" -> 5
),
Map(
"destination" -> "france",
"origin" -> "germany",
"count" -> 10
)
)
This technique isn’t free. It’s slower than .toDF
. But it’s more flexible and readable.
Loading a CSV file
Loading a CSV file with each test isn’t a good idea. It slows down the tests. It also makes the tests less readable. It might be a good idea to load the CSV file once and reuse it in the tests.
A full test
Now a test should look something like this:
val input: DataFrame = createDataFrame(
obj(
"destination" -> "morocco",
"origin" -> "spain",
"count" -> 3
),
obj(
"destination" -> "morocco",
"origin" -> "egypt",
"count" -> 5
),
obj(
"destination" -> "france",
"origin" -> "germany",
"count" -> 10
)
)
val actual: DataFrame = getDestinationsWithTotalCount(input)
val expected: DataFrame = createDataFrame(
obj(
"destination" -> "france",
"total_count" -> 10
),
obj(
"destination" -> "morocco",
"total_count" -> 8
)
)
assertSmallDataFrameEquality(actual, expected, ignoreNullable = true, orderedComparison = false)
The assertSmallDataFrameEquality
function is from spark-fast-tests
library. Check the repository for more examples.
Conclusion
And that’s it. I hope this blog was helpful. If you have any suggestions, feel free to open an issue in the repository.